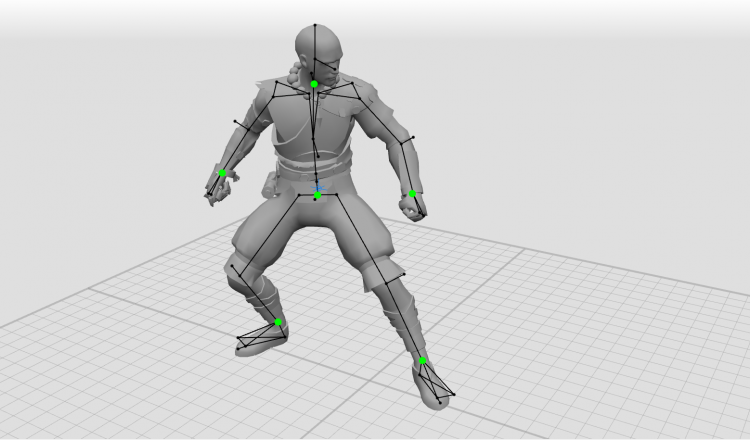
We would like to share with you our first achievements with deep learning in character animation using Cascadeur.
While working on Shadow Fight 3, we accumulated a lot of combat animations - about 1,100 movements with an average duration of about 4 seconds each. Right from the start, we knew that this would be a good data set for training some kind of neural network one day.
During our work on various projects, we noticed, that animators can imagine the character’s pose by drawing a simple stick figure when making their first sketches. We thought that since an experienced animator can set a pose well by using a simple sketch, it will be possible for the neural network to handle it too.
That’s why we decided to take only 6 key points from each pose (wrists, ankles, pelvis, and base of the neck) and check if the neural network can predict the position of the remaining 37 points.
The procedure of the learning process was clear from the beginning: at the start, the network would receive the positions of 6 points from a specific pose, and as an output, it would have to predict the positions of the remaining 37 points.
We would then compare them with the positions in the original pose. In the loss function, we would use the least-squares method for the distances between the predicted positions of the points and the source.
For the training dataset, we had all the movements of the characters from Shadow Fight 3. We took poses from each frame and got about 115,000 poses. But this set was quite specific - the character almost always looked along the X-axis and his left leg was always in front at the beginning of the movement.
To solve this problem, we artificially expanded the dataset by generating mirror poses and randomly rotating each pose in space. This allowed us to increase the dataset to 2 million poses. We used 95% of them for network training and 5% for parameter setting and testing.
The neural network architecture we chose was fairly simple - a fully-connected five-layer network with an activation function and an initialization method from Self-Normalizing Neural Networks. On the last layer, activation was not used.
Having 3 coordinates for each node, we got an input layer of 6x3 elements and an output layer of 37x3 elements. We searched for the optimal architecture for hidden layers and settled on a five-layer architecture with the number of neurons of 300, 400, 300, 200 on each hidden layer, but networks with fewer hidden layers also produced good results.
L2 regularization of network parameters was also very useful. It made predictions smoother and more continuous. A neural network with these parameters predicts the position of points with an average error of 3.5 cm. This is a very high average, but it’s important to take into account the specifics of the job. For one set of input values, there may be many possible output values. So the neural network eventually learned to issue the most probable, averaged predictions.
However, when the number of input points was increased to 16, the error average decreased by half, which in practice yielded a very accurate prediction of the pose.
But at the same time, the neural network could not give out a completely correct pose, while preserving the lengths of all bones and joints connections. Therefore, we additionally launched an optimization process that aligns all the solid bodies and joints of our physical model.You can see the results of this work in our video. These results are quite specific because the training dataset is made of combat animations from a fighting game with weapons. For example, a character is posed as in a fighting stance and turns his feet and head accordingly. Also, when you stretch out his hand, the wrist is turned as if he’s holding a sword.
This led us to the idea of training a few more networks with an expanded set of points that specify the orientation of the hands, feet, and head, as well as the position of the knees and elbows. We have added 16-point and 28-point schemes.
It turned out that the results of these networks can be combined so that the user can set positions to an arbitrary set of points. For example, the user decided to move the left elbow but did not touch the right one. In this case, the positions of the right elbow and right shoulder are predicted in a 6-point pattern, while the position of the left shoulder is predicted in a 16-point pattern.
We believe that this can turn out to be a really promising tool for working with a character's pose. Its potential has not yet been fully realized, but we have ideas on how to improve and apply it for more tasks.